Sam Altman, CEO of OpenAI, recently said that "AI can now see." This bold statement is a testament to the remarkable progress that has been made in the field of multi-modal Large Language Models (LLMs), which is the one of the current leading researching class of AI. These models that are typically trained on massive datasets, usually but not limited text and images. This allows them to learn the relationship between words and the visual world, enabling them to perform a wider range of tasks. In this article, we will discuss some of them and explore the feasibility of each.

Picture: Eye test. Source: Wix.
Multi-modal LLMs and How It Works
Let's start with the reason that inspires this writing. Recently, I came across this paper authored by the Shanghai AI Lab called "Drive Like a Human: Rethinking Autonomous Driving with Large Language Models", identified with 2307.07162.pdf from arxiv.org. In this paper, the researchers propose a novel approach to autonomous driving that leverages multi-modal LLMs.
At the core of this paradigm shift are multi-modal LLMs, an innovative class of AI models that embark on a journey of comprehension by ingesting vast volumes of textual data and images. They meticulously learn to bridge the gap between words and the visual world, seamlessly connecting linguistic constructs with concepts, objects, actions, and even complex scenes. With this profound understanding, multi-modal LLMs are endowed with the extraordinary capacity to perform a multitude of tasks. They can caption images with remarkable accuracy, respond to questions, distill the essence of videos into concise summaries, or weave intricate narratives from the vast canvas of information at their disposal.
The system works as follows: The multi-modal LLM is trained on a massive dataset of images and videos of traffic scenes. Once trained, the LLM is able to generate accurate descriptions of dynamic scenes, articulating the elements within it, such as pedestrians, traffic lights, and other vehicles.
These descriptions are then fed to a separate control model, which interprets this information to make decisions like slowing down, stopping, or changing lanes. The control model also takes into account other factors, such as the speed of the vehicle and the distance to other vehicles.
The advantages of this approach are manifold. Firstly, it obviates the need for labor-intensive hand-crafted features and labels, reducing both costs and time investment. This will indirectly erasing the distinction line between supervised machine learning and non-supervised machine learning as the input class are labelled by non-human. Secondly, it fosters a more natural and human-like form of communication between autonomous vehicles and their passengers or other road users. Thirdly, it offers enhanced adaptability and flexibility, given the ability of multi-modal LLMs to continuously learn from new data and novel scenarios.
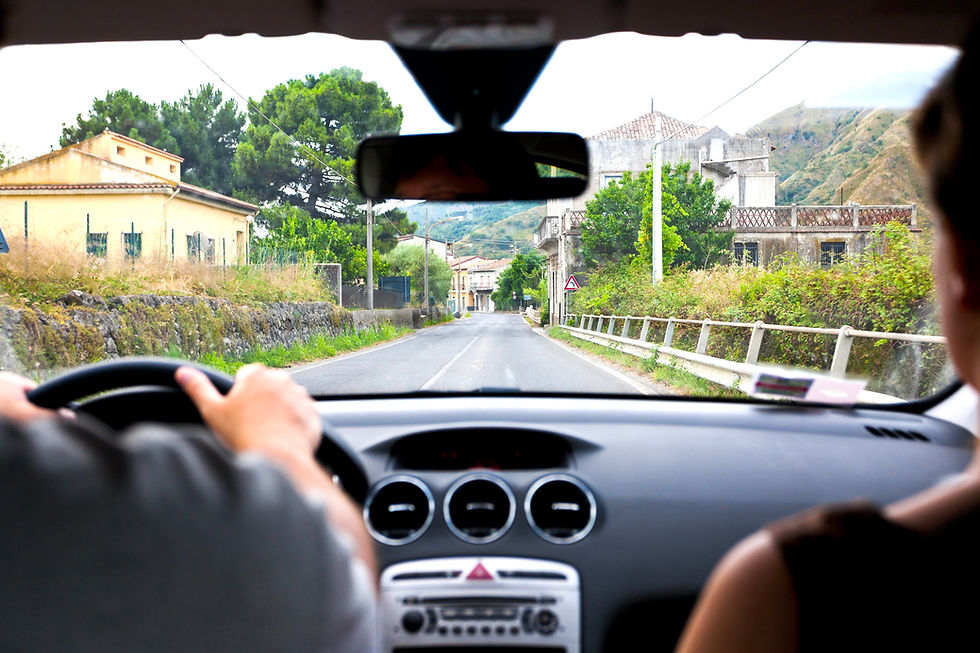
Picture: Self-driving is getting closer. Source: Wix.
Hence, in this context, multi-modal LLMs function as the interpreters of the visual world captured by the sensors and cameras of self-driving cars. They adeptly describe the dynamic scenes, articulating the elements within it, such as pedestrians and traffic lights. These descriptions, in turn, serve as inputs to a separate control model, effectively enabling the car to navigate the road intelligently. This system doesn't merely react to the present; it anticipates future occurrences and charts a course of action accordingly. For instance, the multi-modal LLM may conjure a sentence like, "There is a pedestrian crossing the street ahead, and a red light at the intersection," and the control model interprets this information to make decisions like slowing down, stopping, or changing lanes.
Promising Applications
However, the transformative potential of multi-modal LLMs extends far beyond autonomous driving. Consider these potential scenarios:
Personal Vocal Coaching: Imagine a multi-modal LLM that meticulously scrutinizes your voice and facial expressions to provide constructive feedback on enhancing your singing or public speaking abilities. It becomes a virtual mentor, helping you unlock your full potential at your service all-day suiting to your own schedule, which can be more beneficial to low-income workers who cannot attend day lessons.
Manufacturing Manager and Customized Product Design: In the domain of manufacturing, multi-modal LLMs can serve as vigilant overseers and makers of the production process. By analyzing data from cameras and sensors, they detect defects, errors, or anomalies and communicate these issues in a comprehensible natural language format, facilitating quick corrective actions. Moreover, consumers more often than not prefer to have a variety of choices, and a customized service in some special events. A multi-modal LLM can effortlessly translates your preferences and specifications into a tangible product. It crafts vivid images and detailed descriptions, calculating the required movement and angle of machine (given that its calculation ability increases in the future), then transforming your imagination into reality while optimizing user experience.
Entertainment: In the world of entertainment, multi-modal LLMs become virtuoso content creators. For example, a Youtube video often need a good script and a lot of editing. LLMs can generate a video based on your own scripts, which can reserve a high level of your own personality depending on your own scripts and interaction with the AIs. Even for the music industry, famous signers can license their voices to have their voice in multi-human-language songs, which can increase their global fanbase.
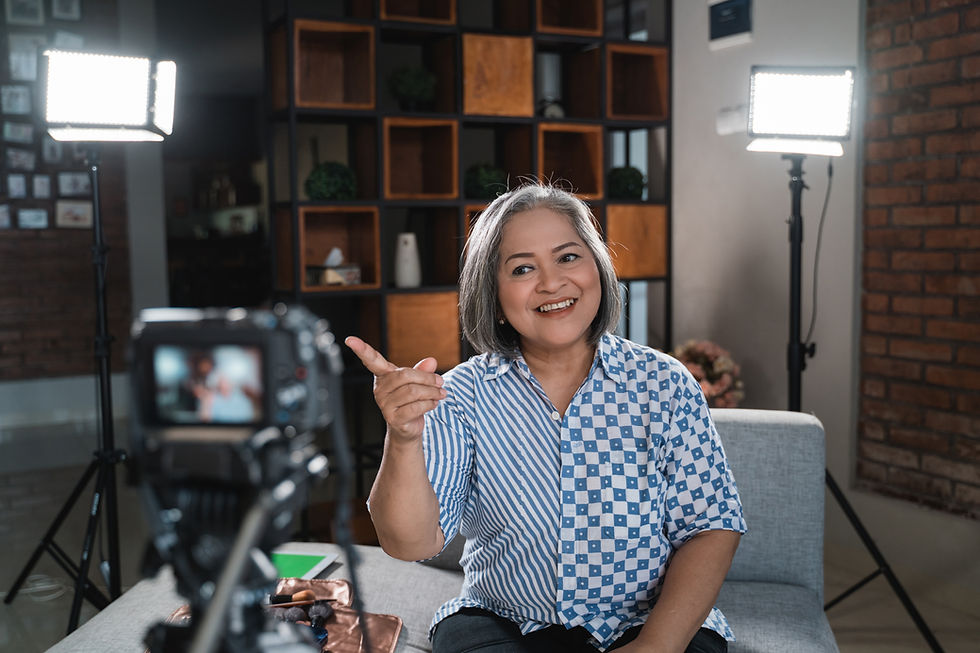
Picture: Making a Video. Source: Wix
Personalized education: LLMs can be used to create personalized educational experiences (more like tutor) for students of all ages. By tailoring their teaching style to the individual needs of each student and providing real-time feedback, LLMs can help students learn more effectively and efficiently.
Architecture: LLMs could be used to help architects design more sustainable and efficient buildings. By analyzing data on weather patterns, energy consumption, and human behavior, LLMs could generate new designs that are optimized for both performance and livability. Embracing the idea of closing the knit between designer and engineering from Elon Musk with his successful implementation in SpaceX and Tesla, maybe architecture, civil engineer and building engineer can be combined or work together more effectively.
Counter Arguments and Feasibility Assessment
From what we just discussed, multi-modal LLMs are a powerful new tool with the potential to revolutionize many industries and aspects of our lives. However, there are also some risks to consider, as well as feasibility assessments of some of the creative applications mentioned above.
Counter Arguments:
Bias: Multi-modal LLMs are trained on massive datasets of text and images, which means that they can potentially reflect the biases that exist in these datasets. For example, an LLM trained on a dataset of images and information relating mostly of white people may be more likely to align to their values and ideas in general. This is a real worry and already happened a few times in the past so it is important to be aware of this potential bias.
Privacy: Multi-modal LLMs need to analyze and generate massive amount of data, which directly means a constant stream of huge data collection. Therefore, users data stored by these multi-modal LLM providers become the biggest treasure trove for bad actors. It is vital more than ever to protect database with higher compliance and security level.
Security: Speaking of security, multi-modal LLMs could also be used as an attacking tool to create new forms of cyber-attacks, such as deep-fakes and phishing emails. It is important to develop security measures to protect against these attacks.
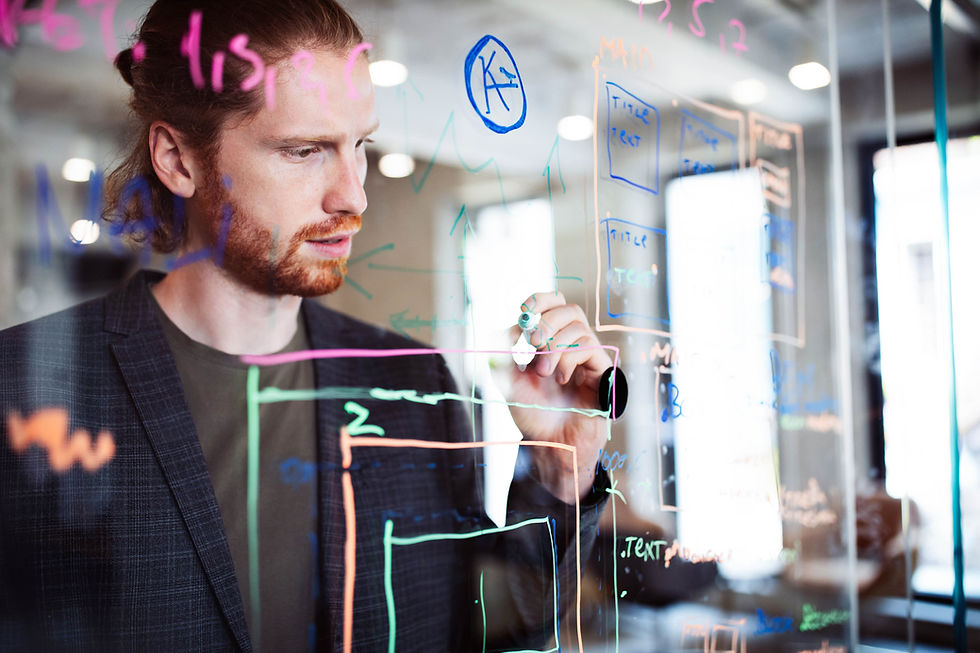
Picture: Thinking. Source: Mix.
Feasibility Assessments:
Personal Vocal Coaching: the hardware requirement for this case is not high, except for GPUs. With a decent-cheap webcam and laptop integrated microphone, the data quality is enough to use. Therefore, the main challenge would be in refining the algorithms and making the tool user-friendly.
Manufacturing Manager and Customized Product Design: The technology for real-time monitoring of manufacturing processes using cameras and sensors already exists. Integrating natural language reporting and product design generation would require software development but is within current technological capabilities. The feasibility would largely depend on the willingness of manufacturers to adopt such systems and the availability of data for model training, as well as designing a new generation of machine to take full advantage of LLMs.
Entertainment: this would require the development of new tools and software for creating and distributing interactive content. It would also require the development of new business models for monetizing this type of content. There are already many tools teasing this exact ability. Hence, the feasibility would depend on the willingness of content creators and platforms to invest in these innovations.
Personalized education: this would require the development of new educational software and platforms that are specifically designed to leverage the capabilities of LLMs. However, the real challenge would be training of teachers on how to use these new tools effectively.
Architecture: Implementing multi-modal LLMs in architecture for design assistance is technologically feasible. The technology to convert natural language descriptions into visual representations exists. However, widespread adoption and merging of disciplines would results in major society changes and potential pushbacks.
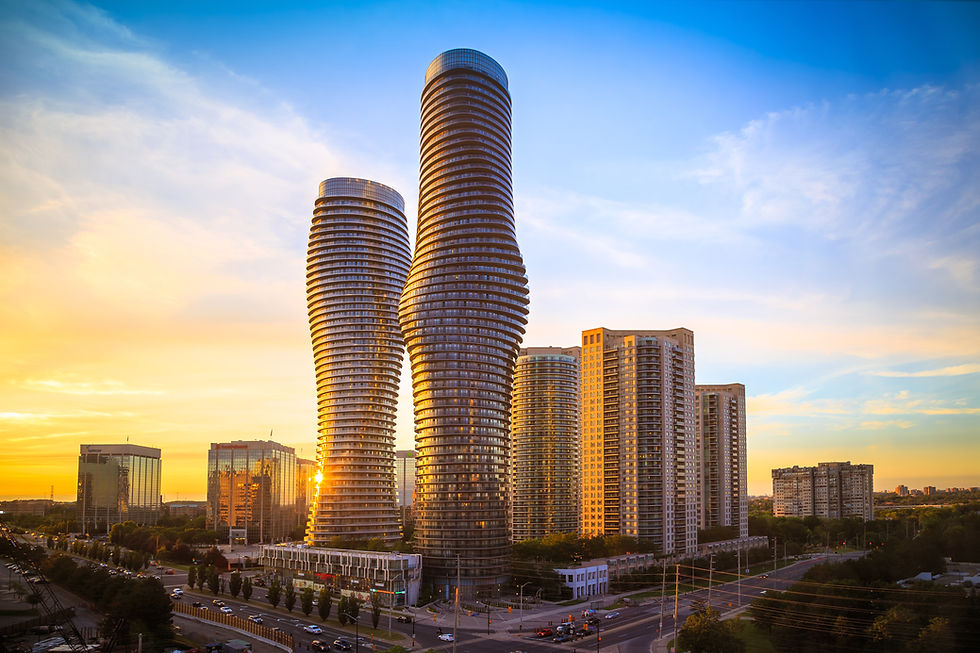
Picture: Bold architectures. Source: Wix
Another bold idea
In the writing of this, I also came across an article about large language models (LLMs) as an operating system for software and systems modeling. It discusses the potential impact of LLMs on the software development process.
The authors hypothesize that LLMs will be used to create specialized modeling languages that can be tailored to specific domains. This would allow developers to focus on the modeling level, while deferring the implementation to an automated generator. The authors also discuss the challenges of developing and using LLMs, such as the need to understand their capabilities and limitations. They conclude by calling for more research on how to use LLMs to improve software development.
In addition to the ideas in the paper, I would like to add that LLMs could also be used to improve the quality of software documentation and enable people with vision or other challanges to code or to simply use computer easily. For example, LLMs could be used to generate documentation that is more comprehensive and up-to-date than traditional documentation. Multi-modal LLMs could also take voice command to execute orders more accurately.
Conclusion
These illustrations merely scratch the surface of multi-modal LLMs' expansive potential. As data continues to flow and research progresses, we can eagerly anticipate an ever-expanding array of awe-inspiring applications. These models are indisputably at the forefront of reshaping our perceptions of artificial intelligence, rendering the once-fantastical visions of human-machine synergy into a tangible, transformative reality.
Comments