The advent of blockchain technology has ushered in a new era of digital transactions, with Ethereum emerging as a prominent player in this transformative landscape. However, as the rise was too rapid, regulations are falling short and this sector became the hottest sector for scammers in recent years. This blog post aims to provide a comprehensive analysis of Ethereum transactions from 2015 to 2023, with a particular focus on distinguishing patterns between legitimate and dodgy classes. In general, there is some clear distinction between legit and dodgy transactions in terms of time of trading and lifetime of addresses.
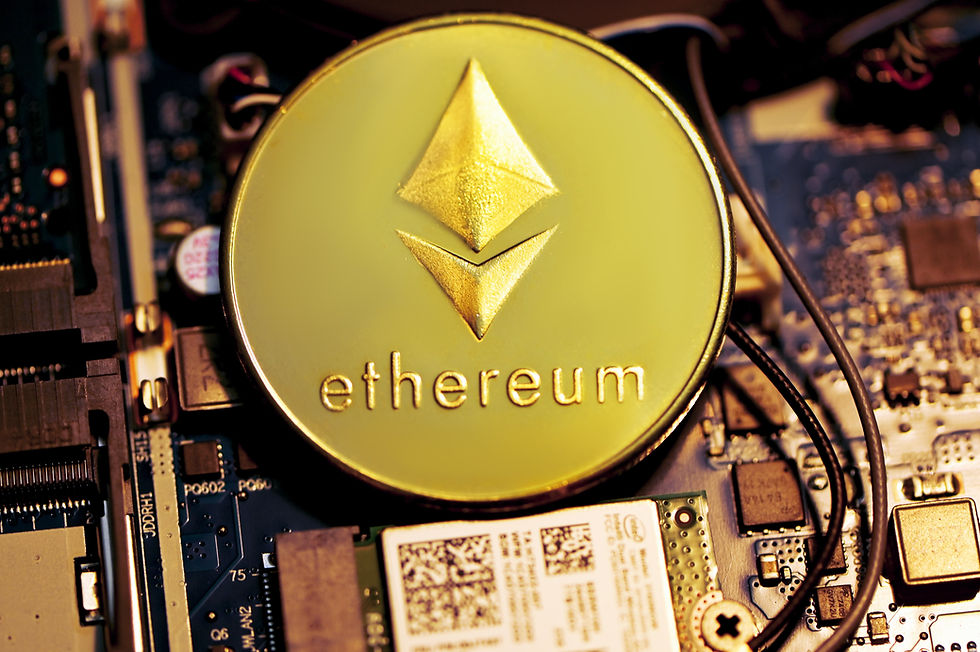
Picture: Ethereum. Source: Unsplash
Data Sources
Before we dive further, it is important to understand the source of information. For this analysis, I took the free 20k Label Ethereum Addresses on Kaggle. This data is taken from EtherScan and CryptoScam.db, which are two credited services for Crypto Inspection. After that, thanks to Google Big Query provides public access to their massive Ethereum transactions and addresses, a comprehensive dataset can be created by merging these transactions with labels from the Kaggle source.
Due to the limitation of free account, I cannot use the full 20k addresses as the file size with full transactions data is too big. However, 10k addresses is already over 10GB with 17M+ transactions.
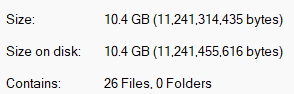
Due to local computational constraint, I used only 4422 addresses for my Machine Learning models, which already gives us 5915144 transactions, which combines with other parameters to create a 5GB csv file.

Picture: Size of Data. Source: Myself.
Deciphering Transaction Patterns
Moving to our first point of discussion revolves around the distinct patterns that emerge when comparing legitimate and dodgy transactions. The data, spanning from 2015 to 2023, indicates that the majority of transactions are conducted by smart contracts, with a substantial portion being legitimate. However, due to limitation of free source, I could not get an even distribution as the data is skewed to 2018-2022. Furthermore, the illegal activities are upto only 2019. Given more financial resource, the premium pro plus package from Etherscan could help with a more even distribution and latest data.
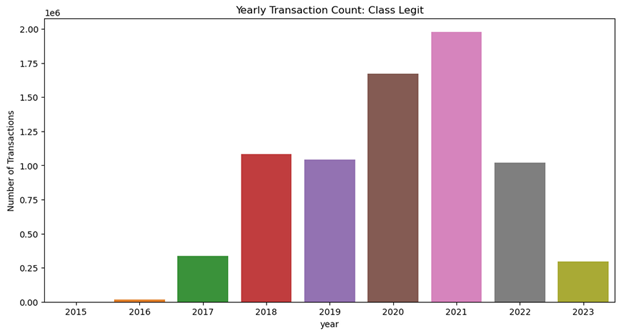
Picture: Data Distribution by year for Legit class. Source: Myself.
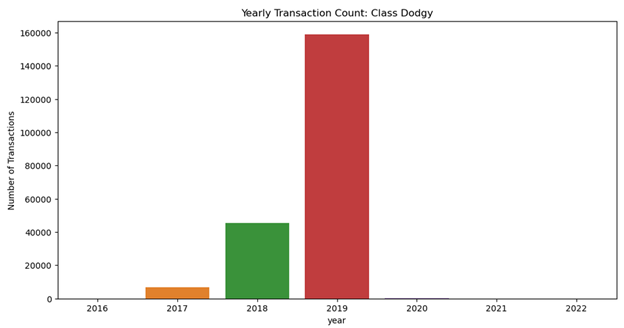
Picture: Data Distribution by year for Dodgy class. Source: Myself.
Notably, as mentioned above, the dominance of "Legit" class is creditable as it is supported by Chain Analysis. In their 2023 crypto review, the ratio between legit and dodgy was approximately 97:3. For our dataset, the ratio is 97:3 as well ('Dodgy': 184144, 'Legit': 5731000). It also has to be noted that majority of transactions are made by smart contract service and not wallet. While this gives more confidence in detecting user dodgy transactions, it can potentially miss out systemic scam crypto projects or scheme likes FTX.
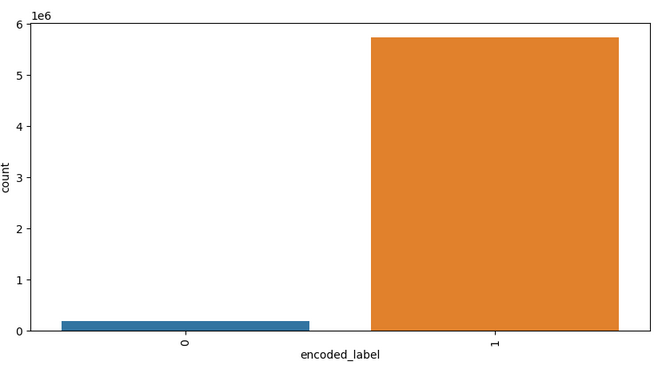
Picture: Majority of transactions are legit. Source: Myself
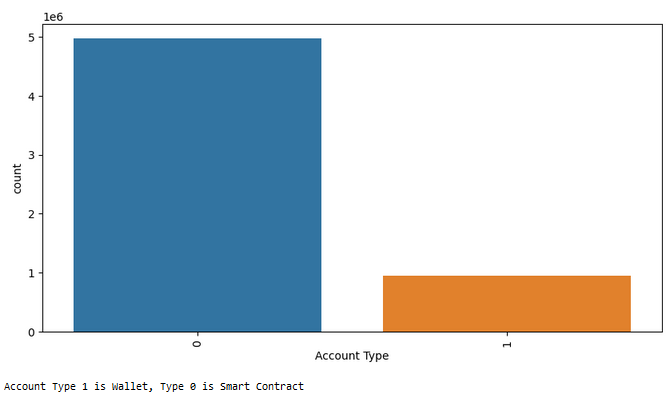
Picture: Majority of transactions are wallets/human made. Class 0 is Smart Contract, class 1 is Wallet. Source: Myself
This observation underscores the trust and reliability that Ethereum has fostered over the years, reflecting its robust security measures and the confidence placed in it by users.
Time Zone and Transaction Classes
The data also incorporates the time zone, specifically UTC–0 (England), providing a global perspective on Ethereum transactions. This consideration underscores Ethereum's global reach and its ability to transcend geographical boundaries. Thanks to its nature, Ethereum can help process cross-border transaction globally faster with less fees than traditional means. Based on the two graphs below, it can be seen that there are clear distinction between legit and dodgy transactions.
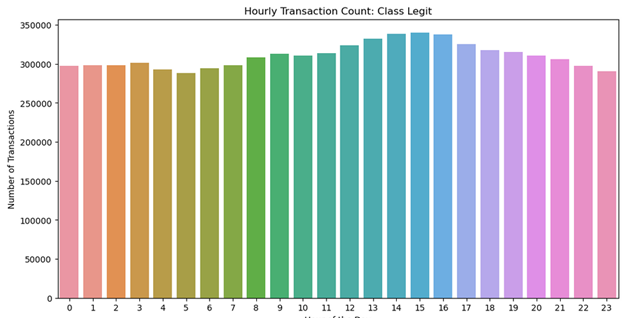
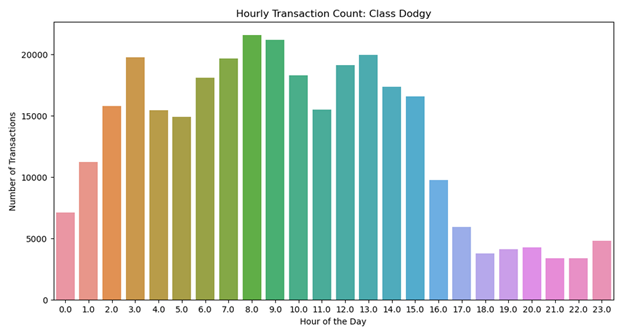
Pictures: Distribution of transaction time time. Source: Myself
In fact, there is a significant drop in dodgy activities volume before 1:00 ( 21:00 UTC-4 Toronto time, 8:00 UTC+7 Bangkok time) and after 15:00 UTC-0 ( 11:00 UTC-4, 22:00 UTC+7). This is not the same case for legit transactions, except for a spike at 8:00 to 9:00 UTC-0 ( 4:00 to 5:00 UTC-4, 15:00 to 16:00 UTC +7). This suggests that there is a correlation between working hours of East Asian with dodgy transactions. Given more resources, we can examine the location of busted illegal activities around the world to verify this correlation.
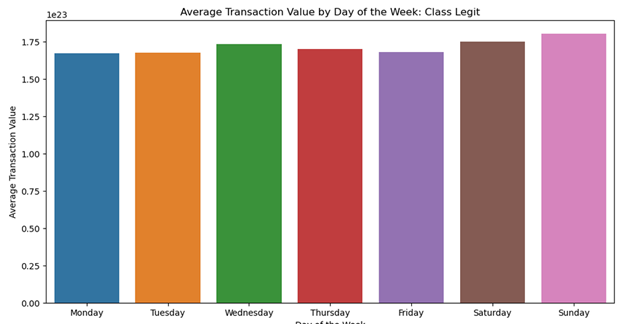
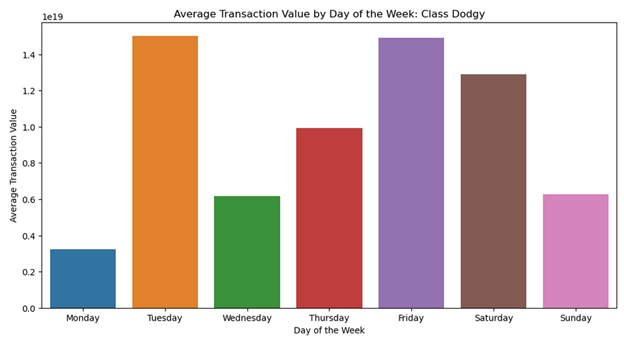
Pictures: Distribution of transaction by day in a week. Source: Myself
It can also be seen that legit crypto trades are done during the weekend as Saturday and Sunday volumes take the top two. On the other hand, bad actors seems to favor Friday, Saturday and surprisingly Tuesday.
Distribution of Transaction Value
Next, we can delve into the distribution of transaction value for each class, represented by two distinct charts. Label 0 corresponds to dodgy transactions, while Label 1 signifies legitimate ones.
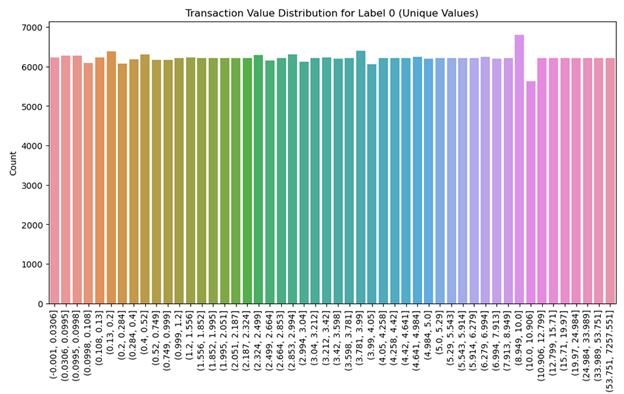
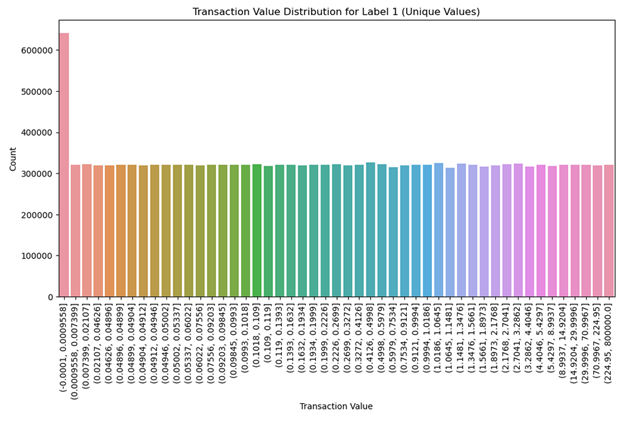
Pictures: Distribution of transaction value. Source: Myself
These visual representations provide a more tangible understanding of the financial implications associated with each class, thereby offering a more comprehensive view of the transaction landscape. In general, the average value of illegal activities are smaller than legit ones. In fact, it is extremely frequent to see bad actors transacted at lower than 0.0009558 ETH, which is less than 2.39 CAD at the time of this writing. This suggests bad actors frequently use mixing services to break big fees into many smaller ones so it is not only harder to trace back but also avoiding auto-fraud detection by crypto exchanges.
Most Active Transactions on Ethereum
As anticipated, the most active transactions occur on the Ethereum platform are from crypto exchanges. This observation is hardly surprising, given that crypto exchange platforms dominate the list.
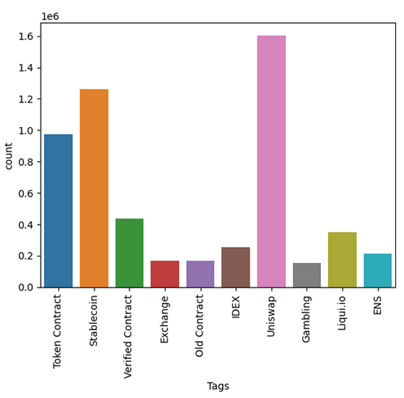
Picture: Most active actors on Ethereum. Source: Myself.
Lifespan of Addresses
An intriguing revelation from the document is that dodgy addresses have a much shorter lifespan than legitimate ones. This observation suggests that illegal addresses are often used a few time before either being blocked or abandoned by bad actors.
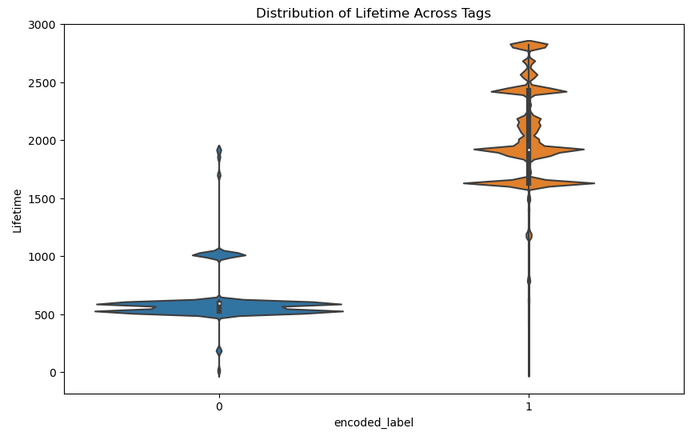
Picture: Distribution of lifetime for Legit (1) and Dodgy (0) classes. Source: Myself
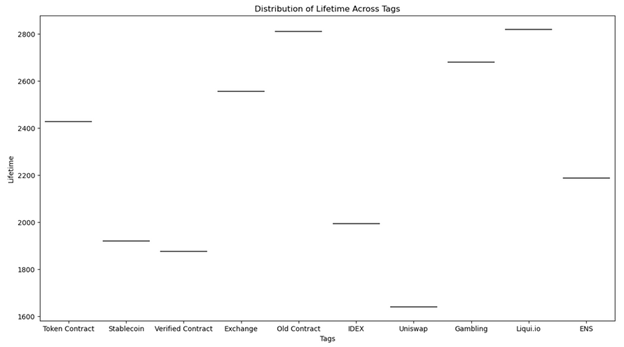
Picture: Distribution of lifetime for most popular tag classes. Source: Myself
Please be noted that the y axis unit are days. As can be seen from the chart, there is an abnormal sign at 1000s days for dodgy. This suggests the nature of crimes are different, where accounts can be hacked or stolen, which it effectively changes label from legit to dodgy. The below table is a sample for distribution of illegal activities type.
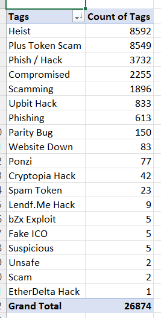
Picture: Distribution for illegal activities types. Source: Myself
Cluster by Tag Analysis
Finally, I present a cluster by tag analysis. This analysis categorizes addresses based on their tags and examines their differences based on the number of withdrawals and deposits. This approach can provide a more granular understanding of the transaction activities on the Ethereum platform.
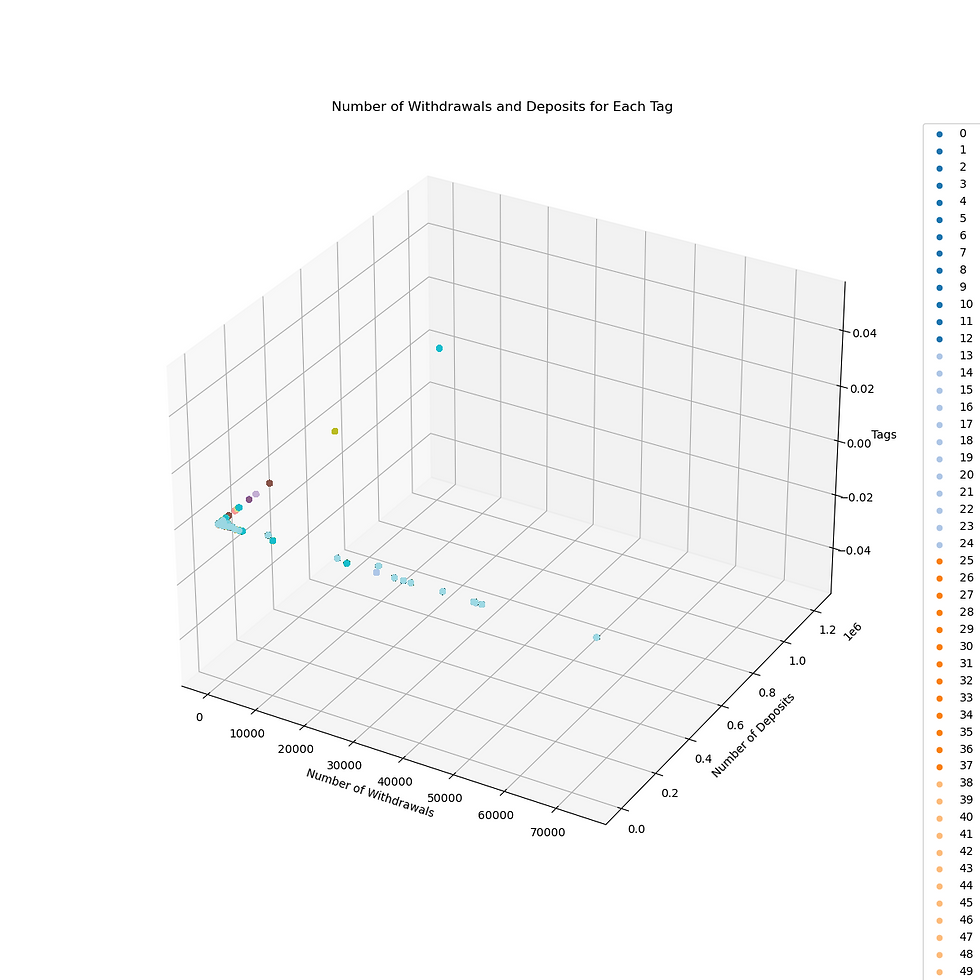
Picture: Clustering tags by number of deposits and withdrawals. Source: Myself
It has to be understood that there are hundreds, if not thoundsands, type of actors on Ethereum. For this dataset, we obtained 200+ tags for different actors. In this graph, the tags number from 220 dominates the chart and overlapping other clusters. Hence, I proceed to categorize tags based on the general sentiment usually associated with the terms. For instance, terms like 'Scam', 'Scamming', 'SpamToken', 'Suspicious', 'Unsafe', 'UpbitHack', and 'bZxExploit' are typically associated with negative situations or activities, thus they are categorized as negative. On the other hand, terms like 'Security', 'Stablecoin', 'VerifiedContract', 'WhiteHatGroup', etc. suggest safe or positive activities, hence they are categorized as positive.
Important Note: Bad actors using positive/legit crypto exchanges need more data for analysis. ALSO, bad crypto exchanges like FTX need time to uncover.
The detail of sentimental categorization:
a) Positive Tags: Trading, Travel, TrustToken, Uniswap, Unstoppable Domains, Upbit, VPN, VR, Vehicle, Verified Contract, Wallet App, White Hat Group, Wi-Fi, YUNBI, ZB.com, Zapper.Fi, dYdX, mStable, tBTC
b) Neutral Tags: Vehicle, VR, VPN, Unstoppable Domains, TrustToken, Trading, Travel, Zapper.Fi, ZB.com, YUNBI, Wallet App, Wi-Fi
c) Negative Tags: Unsafe, Upbit Hack, Website Down, bZx Exploit
The tag "nan" does not carry sentiment as it typically stands for Not a Number or missing value in data science terminology.
The ratio calculation becomes:
Positive : Negative : Neutral = 18 : 4 : 12.
This sentiment-based categorization of tags provides a unique perspective on the nature of activities on the Ethereum platform. It also reiterates that majority of actors on the exchange are more likely to be good actors, which is contrast to mainstream media belief.
Conclusion
This comprehensive analysis of Ethereum transactions provides valuable insights into the patterns, distribution, and activities on the platform. The cluster by tag analysis provides a unique perspective on the nature of transactions, highlighting the importance of sentiment in understanding the potential risks and opportunities associated with different types of transactions. As we move forward, it will be interesting to see how these patterns evolve and what they mean for the future of Ethereum and blockchain technology.
The analysis also underscores the potential risks associated with blockchain transactions, particularly in the context of dodgy transactions. For instance, it seems to be some correlation between dodgy transactions being made in Asian working hours. Thus, it highlights the need for continuous vigilance and enhancement of security measures to ensure the continued trust of users.
Comments