Comparing the Performance of the Newest AI Models: Claude 2.1, GPT-4 and More
- JHN
- Nov 22, 2023
- 4 min read
Artificial intelligence (AI) models are becoming more powerful and capable of generating impressive natural language texts. However, how well do they perform when it comes to recalling information from long documents? In this blog post, I will compare quickly the recall ability of the two newly high-end AI models, Claude 2.1 and GPT-4 128k, based on their ability to answer questions about randomly inserted statements in various documents. The analysis was done by Greg Kamradt, a researcher and AI enthusiast. Then, we will see some interesting development with smaller models. For more detail, please visit the original sources.
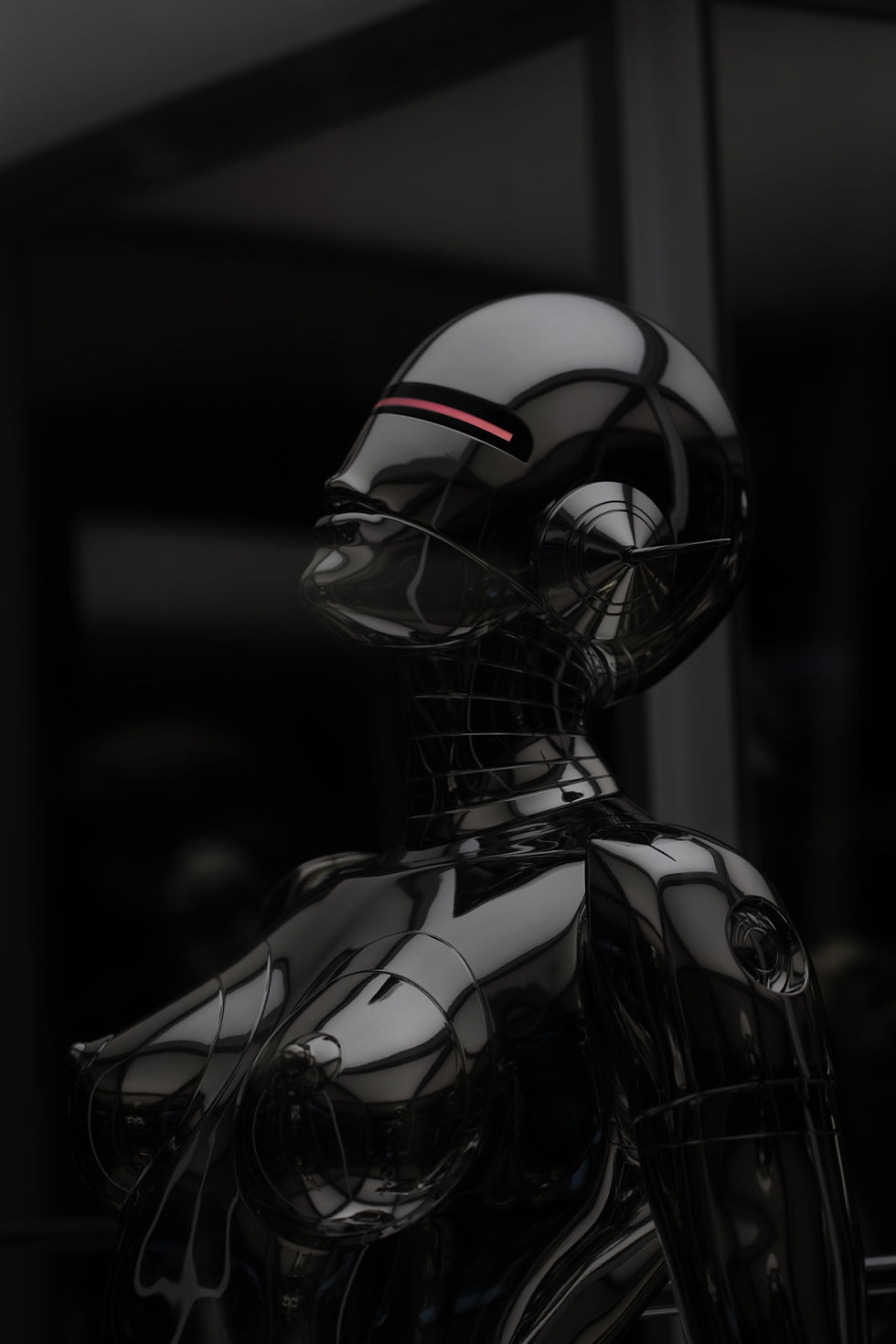
Figure: AI. Source: Unsplash
Large Models Performance
Claude 2.1 is a large-scale language model that has 200k tokens, which is equivalent to about 470 pages of text. It claims to have a high capacity for long-term memory and reasoning. To test its performance, Kamradt placed random statements at various positions in 35 documents with different lengths, and then asked Claude 2.1 to answer questions about those statements. The results showed that Claude 2.1 could recall well the facts that were at the top and bottom of the document, but its performance started to decline from 90k tokens onwards. The cost of using Claude 2.1 was about $8 per million tokens.
GPT-4 128k is another large-scale language model that has 128k tokens, which is equivalent to about 300 pages of text. It is based on the GPT-3 architecture and claims to have improved natural language understanding and generation. Kamradt used the same method to test its performance as he did for Claude 2.1, however with fewer documents and lengths. The results showed that GPT-4 128k had a similar pattern of recall as Claude 2.1, but its performance worsened from 73k tokens. Moreover, it had a lower recall for the statements that were in the early to mid position (7-50%) of the document, while it was also good for the top and bottom positions. The cost of using GPT-4 128k was about $1.28 for 128k input, which translates to about $12 for one million tokens, making it more expensive than Claude 2.1.
Based on this analysis, we can see that both Claude 2.1 and GPT-4 128k have some strengths and weaknesses when it comes to recalling information from long documents. They both perform well for the facts that are at the extremes of the document, but they struggle with the facts that are in the middle or beyond a certain length threshold. This suggests that they have limitations in their long-term memory and attention mechanisms, and that they may need further improvement to handle longer and more complex texts. The cost factor is also an important consideration, as GPT-4 128k is more expensive than Claude 2.1 for the same amount of input.
Furthermore, based on various example on X, formerly Twitter, it seems Claude achieves 2x lower hallucination rate mainly due to rejecting to answer when it detects sensitive words. For instance, when it detects the sensitive K word below, it refuses to give an answer. This refers to the trade-off between usefulness and safe AI, where a useless model can be 100% safe when refusing to answer majority of questions.

Picture: Claude refuses to answer simple questions. Source: X
Small Models Trends
Orca, a Small Language Model (SLM), introduced by Microsoft just released their second generation. Although with only 13B, it shows promising sign of outperforming the Llama-70B model and even Llama 2 13B on certain benchmarks (picture below). Although it can only mostly outperform the legacy version of Llama LLM model, the Orca 2 paper suggests a proven path for training smaller models by using multiple techniques, including step-by-step, recall then generate, recall-reason-generate and direct answer. Depends on the task, the model will automatically choose the suitable strategies to infer.
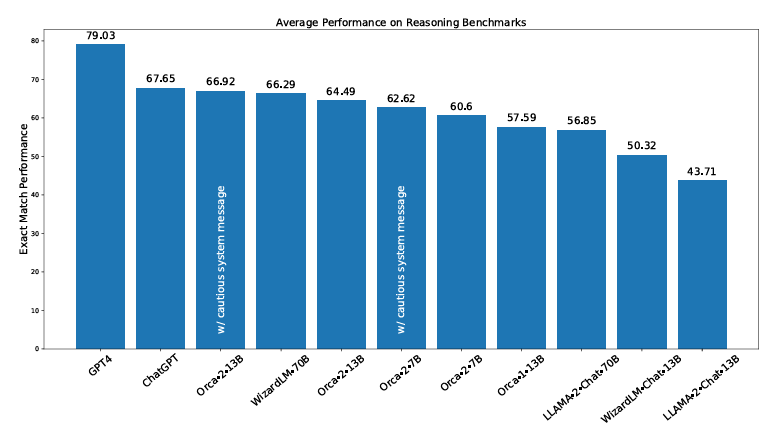
Picture: Orca 2 performance compared to other models. Source: Microsoft.
However, as Jim Fan, a former OpenAI intern and currently Nvidia employee, posted on social medias, the traditional benchmark for LLM are highly deceptive and unreliable. Furthermore, performance of LLMs in the wild with public access tends to show a very different result, especially in term of learning areas not covered in the training datasets. Hence, while this paper demonstrates potential use cases for smaller models, we need to take the benchmark evaluation with a pinch of salt.
Conclusion
In conclusion, this blog post has presented a performance assessment of two newly high-end AI models, Claude 2.1 and GPT-4 128k, based on their ability to recall information from long documents. The analysis was done by Greg Kamradt using a novel method of inserting random statements in various documents and asking questions about them. The results showed that both models have some advantages and disadvantages in terms of recall, memory, attention and cost. Furthermore, the trade-off between hallucination and usefulness are clearly evident in the case of Claude 2.1.
Regarding smaller models, smaller models like Orca 2 are making a path for smaller models to compete with larger ones. In fact, the training technique for smaller models might inspire new learning path for larger models in the future. This blog provides some insights into the current state of the art of natural language generation and understanding, and also raises some challenges and opportunities for future research and development.
Comments